NoStrandAMust
Learning Ground Interaction Models to Increase the Autonomy of Mobile Robotic Exploration Systems

In the project, data from different robots on different soils will be recorded in order to learn soil interaction models with them. These will be integrated into a simulation environment and then used for autonomous adaptation of path planning or locomotion to increase the safety and efficiency of the robots.
Project details
In order to achieve the overall goal of the joint project, the autonomy of robotic systems by ground interaction models, four essential contributions are made in NoStrandAMust four essential contributions are made:
- Different systems here refers to different locomotion modes, propulsion systems, weight classes, and contact geometries and materials. Data will be collected from six walking systems with different morphologies and foot designs, as well as data from different wheel-driven concepts, each of which will be tested on four different surfaces under circumstances with different locomotion behaviors.
- state-of-the-art regression and classification methods as well as other machine learning methods are required for the generation of soil interaction models. In particular, preliminary work with artificial neural networks has shown that they are very well suited to represent models comparable in complexity to those required in this project.
- By integrating the models into a simulation environment, they can be evaluated based on the holistic robot behavior and compared with the behavior from the data acquisition of the real systems. The goal is an accurate real-time simulation that can be used for system design as well as path planning.
- by continuously matching robot performance with a prediction generated from a simulation running on the robot, the systems will be able to continuously determine soil conditions and detect deteriorating conditions at an early stage. The robot should react accordingly to changing soil conditions, for example by triggering new path planning or appropriate behavior adjustments.
Since the data-driven approach means that the soil interaction models have to be trained on a system-specific basis, it is not possible to generate a generally applicable model in NoStrandAMust, as is possible with analytical models. Nevertheless, in order to demonstrate the general applicability of the method, six different systems will be used in NoStrandAMust. These include three walking machines (Charlie, CREX, and Mantis), two wheel-driven systems with different concepts (SherpaTT, and Artemis), and a mixture between walking and wheel systems in the form of a so-called star wheel (Coyote III). In addition, the weight class of the systems ranges from about 20 kg to about 120 kg for both the running systems and the wheel concepts. Each of the six systems is tested on substrates with different properties. In addition to a distinction between loose sedimentary soils and hard subsoils, there will also be different variants within these two classes. It is envisaged here that the ''loose sediment'' class will include fine sand, fine gravel, coarse gravel or basalt split. For hard soils, the systems will be used to record data that have different friction coefficients, such as dust-covered surfaces or high friction as found in lava caves.
Videos
ARTEMIS: First test run with a penetrometer
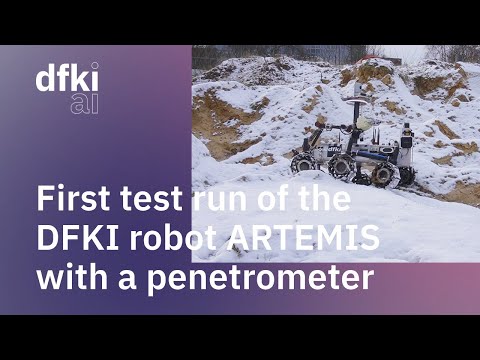
The rover Artemis, developed at the DFKI Robotics Innovation Center, has been equipped with a penetrometer that measures the soil's penetration resistance to obtain precise information about soil strength. Such measurements allow for conclusions about the current soil condition, applicable in both agricultural settings and space exploration. The video showcases an initial test run with the device mounted on the robot. During this test, the robot was remotely controlled, and the maximum penetration depth was limited to 15 mm.